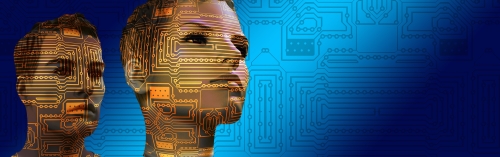
Overview
Artificial intelligence (AI) enables machines to simulate human intelligence. Machine Learning is a subset of AI that includes the ability to learn as it is constantly fed data. Another subset of AI is reasoning, which refers to an artificial intelligence system’s ability to solve problems or draw conclusions.
We will discuss how AI works in layman’s terms, and we have posted Technical Notes for those who want to delve further into it. If you just want the basics, you can skip those parts.
A Little Background on Computer Programming
Writing a Computer Program
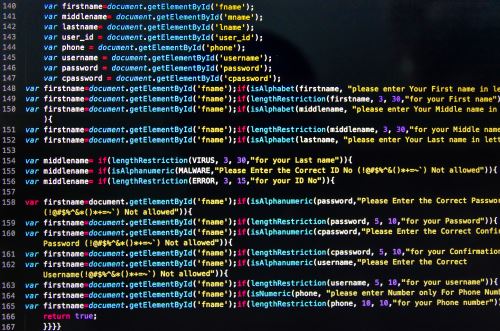
Our bits and bytes article discussed how computers are programmed using instructions, but let’s recap as a refresher!
Computer programs tell the computer to do things by reading instructions that a human programmer has entered. One of our examples was a program that distributes funds to an ATM recipient. It was programmed to distribute the funds if there was enough money in the person’s account, and not if there wasn’t.
But THIS IS NOT AI since the instructions are specific and there are no variations to decide anything other than “if this, then that”.
In other words, the same situation will occur repeatedly with only two results. There is no determination that there may be more aspects to just delivering money, such as determining the potential for fraudulent activity.
Bottom line – There is no learning involved.
Enter Artificial Intelligence – A Simplified Representation
You are a robot, but like the Scarecrow in The Wizard of Oz, you have no brain. John, the human, wants to fill your brain with knowledge, so he starts by showing you a picture of a fire engine.
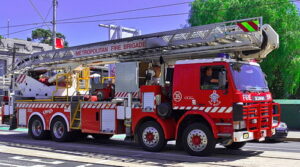
But to the robot, it’s just a bunch of pixels (those tiny dots that make up an image on a computer screen). In AI terms, it is called raw data. This raw data of pixels means nothing to your brain. So John helps by labeling different features of what makes up the fire engine. Things like the ladder, hose, color, tires, and emergency lights, to name a few.
As mentioned, a feature represents one part of the fire engine, such as the ladder, hose, color, tires, and emergency lights. If this were a table, the feature would be the column. Another way of saying it is that the feature is a piece of information that describes one of the object’s components.
The actual information or data for each feature is called the feature value. Under the color feature would be the data ‘red’, and under the hose feature, the data would say ‘yes’. It would be one of its cells if this were in a table.
All these features form a complete data point defining the entire fire engine image.
As your brain sees more examples (data points), it notices patterns in the different characteristics of the objects. Eventually, it learns to differentiate what each object presented to it as input is.
A fire engine would have a row of feature values that describe it, an ambulance would have its own row, and a Chevrolet Corvette would also have its own set of feature values. Each row is a data point, and the complete set of data points is a ‘dataset.’
Labeling the features is called ‘supervised learning.’
Visualizing Feature Values, Features, and Datapoints Using a Table
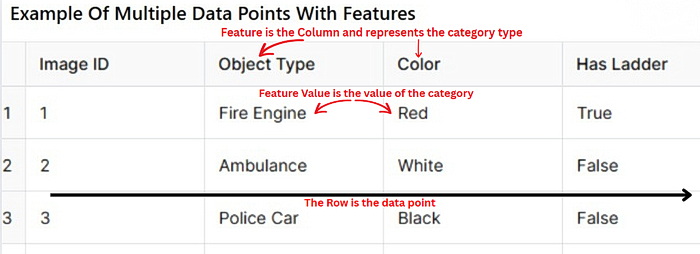
📊 Another Example:
Columns are the features. Rows are the datapoints, and the table is the dataset that contains datapoints of different houses.
ID | Sq Ft (feature) | Bedrooms (feature) | Year Built (feature) |
---|---|---|---|
1 | 2000 | 3 | 1995 |
2 | 1500 | 2 | 2001 |
The entire table = dataset
Each row = datapoint
Each column = feature (what category of information is in that column)
Each cell = a value of the feature
Now, you have the data necessary to identify a fire engine, an ambulance, and a police car. Know what parts it encompasses and how to distinguish it from other vehicles.
Once the datapoints are identified and the AI can recognize them to conclude what the raw data is, it is no longer raw data and becomes an ‘entity‘.
Congratulations! You are now a machine that can differentiate between objects. More specifically, you have the materials available for a program to read and determine what kind of vehicle it is.
Reviewing the Terms
Raw Data
Feature
Feature Value
Datapoint
Dataset
Entity
Supervised Learning
Now that we understand how data is organized — with features, feature values, and data points – let’s talk about how a machine learns from all of this.
This is where deep learning begins.
Deep learning is a special type of machine learning. There is more than one type of machine learning, but we’ll focus on this one that uses a neural network—a system inspired by how the human brain processes information.
Each time the machine sees a new image or piece of data, it uses what it has already learned to make a prediction, and then it adjusts itself based on whether that prediction was right or wrong.
The basic premise behind AI is to create algorithms that scan unknown data (input data) and compare it to data it has already learned from the dataset. So, let’s start by looking at an example.
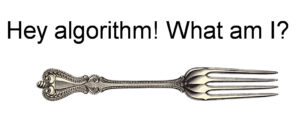
The AI Mindset
Imagine I’m a generative AI, and you show me a picture of a fork.
I ask myself: “Is this a fork or a spoon? Maybe a knife?” I notice all three have handles, but this one has spikes—that’s a clue. I look at my notes I have written about everything I’ve learned so far. These notes in AI vernacular are called internal parameters. Some of these parameters match the features I see in the picture (the input.) Some don’t.
So I make a prediction: “I think this is a knife.”
Then, I call my friend to verify by looking at the dataset and determining if I am correct. My friend is called the loss function. He goes to another room where the dataset resides, as I’m not allowed to go into that room.
If I’m right, great! But if I’m wrong, my friend comes back and says, ” Nope—try again. And this is how wrong you were (by providing a number in a weighted format representing the amount off I am.
But before I try again, I need another friend –backpropagation, whose function is to tell me how to update my notes (internal parameters) so I can get it right next time.
And just like that, I learn a little more with every mistake.
AI algorithms scan the characteristics of unknown data, called patterns. They then match those patterns to data they already recognize, which is called pattern recognition. The data they recognize is called training data. The result is that they decide what that unknown item is.
The patterns within the dataset are called features. Also called machine learning, it is the whole process of scanning, comparing, and determining. (There are seven steps involved in machine learning, and we will touch upon those steps in our article on artificial intelligence 102.)
If you write a computer program that allows you to draw a kitchen on the screen, you would need a dataset that contains features representing the different items in the kitchen, such as a stove, fridge, sink, and utensils, to name a few. The program would then need to access and assemble the components on the screen; hence, our analysis of the fork in the image above.
Note: The more information (features) input into the dataset, the more precise the algorithm’s determination will be.
Supervised Learning
Examining and comparing a particular object to the object you possess by reading the labels of the components in the dataset and assembling them.
Example: Consider a dataset like a recipe book containing labeled ingredients and instructions. Each feature tells the AI algorithm what something is — for example, “this image is a cat” or “this sentence is a question.” The AI reads all these examples to learn how to make predictions. The more features a dataset has, the better AI recognizes and creates new “recipes.”
Writing a Learning Program
The ATM example is limited to two options, but AI is much more intensive than that. It is used to scan thousands of data items to conclude.
How Netflix Does It
Have you ever wondered how Netflix shows you movies or TV shows that are tuned to your interests? It does this by examining your preferences based on your previous viewings.
The algorithm analyzes large amounts of data, including user preferences, viewing history, ratings, and other relevant information, to make personalized recommendations for each user.
It employs machine learning to predict which movies or TV shows the user will likely enjoy.
It identifies patterns and similarities between users with similar tastes and suggests content that has been positively received by those users but hasn’t been watched by the current user.
For example, suppose a user has watched science fiction movies. In that case, the recommendation might be to suggest other sci-fi films or TV shows that are popular among those users with similar preferences.
The program will learn and adapt as the user interacts with the platform, incorporating feedback from their ratings and viewings to refine future recommendations.
By leveraging machine learning, streaming platforms like Netflix can significantly enhance the user experience by providing tailored recommendations, increasing user engagement, and improving customer satisfaction.
This can’t be done using the non-learning ‘if-else’ instruction program we previously spoke about in the ATM example.
A Gmail AI Example
As you type your email, Google reads it and then offers words to accompany the sentence that would coincide with what you are about to type before you have even typed it.
This is called language modeling, which uses the Natural Language Processing (NLP) model.
In NLP, the algorithm uses a probability factor to predict the most likely next word in a sentence based on the previous entry.
AI algorithms feed on data to learn new things.
The more data (data points) that exist, the easier it will be for the model to identify the patterns of an unknown entity.
AI: How It All Works
There are three main types of machine learning: supervised learning, unsupervised learning, and reinforcement learning.
Click CC above for closed caption
Supervised Learning
This is the most common type of machine learning. It involves feeding a computer a large amount of data to recognize patterns from the labeled dataset and make predictions when confronted with new data.
In other words, supervised learning involves training a computer program to read from a data sample (dataset) to identify unknown data.
How the Machine Thinks with Supervised Learning
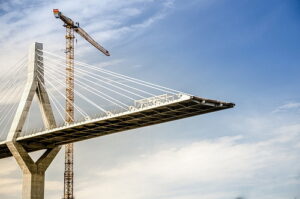
Show and Tell: A human labels a dataset with data points, identifying the sample set as a building.
Then, humans do the same thing to identify a bridge. This is another classification, different from the building classification, and is identified with specific patterns that make up a bridge.
The program takes note of the patterns of each classification. If computer instructions were written in plain English, this is what it would say:
This is a bridge. Look at the patterns that make up the bridge. And this is a building. Look at the patterns that make up the building. They both have some standard components (e.g., Steel and concrete), but I can also see distinguishable differences. Let me match them up to the data I just received (input data), compare it to my dataset of components, and decide whether this new data is a bridge or a building.
Supervised learning is used in many applications, such as image recognition, speech recognition, and natural language processing.
Supervised learning uses a data sample to compare unknown data. The data sample is called a data model.
It’s Raining Cats and Dogs
A supervised learning algorithm could be trained using images called “cats” and “dogs”; each cat and dog is labeled with data points that distinguish each.
The program would be designed to learn the difference between the animals using pattern recognition as it scans each image.
A computer instruction (in simplified terms) might be “If you see a pattern of thin lines from the face (whiskers), then this is a cat”.
The program would then be able to distinguish whether the new image it presents is that of a cat or a dog!
This type of learning involves two categories – cats and dogs. When only two classifications are involved, it is called Binary Classification.
Supervised Learning Using Multi-Classifications
An Example
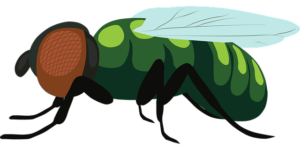
Suppose you are studying insects and want to separate flying insects from crawling ones. Well, that’s easy. You take a bug you found in your backyard and compare it to the ant and fly you already stored on your insect board. In AI terms, this is supervised binary classification.
You immediately know, based on the pattern configuration of the insect which classification it belongs to – the crawlers or the flies. Now you grab more flies and put them in the fly category and do the same with the creepy crawlers for their category.
Let’s say you want to go deeper in the fly classification and find out what type of fly it is (e.g., house fly, horse fly, fruit fly, horn fly, etc.), but you only have two classifications to compare them two – flies and crawlers, so what do we do? You create more classifications for the fly class.
These are multi-classifications, or multi-class classifications, which provide additional labeled classes for the algorithm to compare the new data to.
We will delve more into multi-class classifications and how this works in our next article, but for now, just know what binary classifications and multi-class clarifications are.
Unsupervised Learning
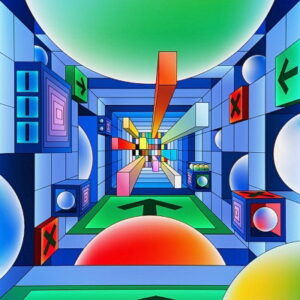
Unsupervised learning involves training a computer program without providing any labels or markings to the data. The aim is to enable the program to find (learn) patterns and relationships on its own.
It does this by reading millions of pieces of information and grouping them into categories based on their characteristics or patterns. Then, it decides what the new entity is by matching it up to one of those categories.
In other words, it matches patterns of the unknown data to the groups it created and then labels them without human intervention. This is called clustering.
Anomaly detection is identifying data points that are unusual or different from the rest of the data. This can be useful for tasks such as fraud detection and quality control.
Reinforcement Learning
Reinforcement learning (RL) learns by trial and error, receiving feedback as rewards or penalties for its actions. Any negative number that gets assigned means it is punished.
The higher the negative number, the more the algorithm will learn not to pursue that particular circumstance and will subsequently try again until positive numbers are assigned, which is called a reward. It will continue this process until it is appropriately rewarded. RL aims to maximize its rewards over time by finding a sequence of actions that leads to the highest possible reward.
One of the defining features of RL is the use of a feedback loop in which the agent’s actions (an agent is the decision-making unit responsible for choosing actions in the environment provided to it). The loop lets the agent learn from experience and adjust its behavior accordingly.
The feedback loop works as follows:
- The agent takes an action in its environment.
- The environment gives the agent feedback about the action, such as a reward or punishment.
- The agent then updates its policy based on the input.
- The agent will repeat steps 1-3 until it learns to take actions that lead to desired outcomes (rewards).
RL has been applied to various problems, such as games, robotics, and autonomous driving. It is handy in scenarios where the action may not be immediately evident and where exploration is necessary to find the best solution.
Conclusion
Overall, these AI methods are widely used in various industries and applications. As artificial intelligence technology advances, we will continue to see growth and development.
What are the advances or dangers that AI can bring to the future? Please read our article on the pros and cons of AI to learn more.
Machine Language Terms to Know
- Computer Instruction
- Computer Program
- Algorithm
- Data Points
- Patterns
- Labeled Data
- Dataset
- Data Model
- Pattern Recognition
- Machine Learning
- Binary Classification
- Multiclass Classification
- Supervised Learning
- Unsupervised Learning
- Reinforced Learning